Near-Term Machine Learning Use Cases for Hospitality
12 experts shared their view
Using interfaces to access the multitudes of information housed within a hotel's property management system (PMS), technology vendors have on their hands a literal treasure trove of first-party training data for machine learning applications. Any primary data extraction must nevertheless be focused around specific use cases that must be tested and verified before deploying in a live environment. Thus far, we've seen lots of discussion around what's possible in the abstract for machine learning, but outside of perhaps chatbots and algorithmic rate optimization recommendations, many of the purported use cases are not practical for the here and now.
So, if we look specifically at machine learning applications based upon a PMS data extraction, what top three use cases or tools should hoteliers investigate in 2024, and what tangible value will be created?
HN Thematics
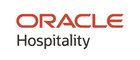
This World Panel Viewpoint is sponsored by Oracle Hospitality
More information
GenAI Copiloting Use Cases for Hoteliers Applicable Today
Using interfaces to access the multitudes of information housed within a hotel's property management system (PMS), technology vendors have on their hands a literal treasure trove of first-party training data for machine learning applications. Any primary data extraction must nevertheless be focused around specific use cases that must be tested and verified before deploying in a live environment. Thus far, we've seen lots of discussion around what's possible in the abstract for machine learning, but outside of perhaps chatbots and algorithmic rate optimization recommendations, many of the purported use cases are not practical for the here and now. So, if we look specifically at machine learning applications based upon a PMS data extraction, what top three use cases or tools should hoteliers investigate in 2024, and what tangible value will be created?
There are some uses cases that would be very interesting to deep dive with machine learning applications to unlock valuable insights and improve efficiencies for hoteliers.
A first use case should be targeting guest personalization. This use case will benefit mainly upscale and luxury hospitality. Machine learning algorithms could analyze Guest’s data such as booking history, billing details, preferences. The outcome and tangible value would be the possibility to define patterns for next reservation for either a specific guests or a specific context. This use case would be more efficient and pertinent when it concerns a large volume of hotels with a unique guest profile management between them.
Another use case would bring benefits in helping automatize administrative task like room’s status reconciliation. By analyzing historical data and different context of room occupation (business traveler, family stay, etc.), room’s status change could be predicted thus helping the housekeeping team define their priority list for cleaning.
Last but not least, analyzing historical occupancy data and retrieving major events happening in the city or region from a Revenue Management system (for example), Machine Learning could help plan staff schedule in the hotel for mid and long visibility.
Machine learning is particularly well-suited for tasks that involve analyzing large volumes of guest data to identify patterns, trends, and non-obvious relationships. This can extend from enhanced guest experiences through streamlined check-in processes, to more personalized experiences that empower employees to allocate more time to value-driven tasks.
Oracle has exposed the largest set of API on the market through the Oracle Hospitality Integration Platform (OHIP). Combining access to guest and transactional data with these API's, customers and partners can create AI and machine learning innovations that elevate guest experiences, simplify operational tasks, and refine staffing efficiency.
Hotels and technology partners can create and test these new AI technologies faster and cheaper than ever before. Where it previously may have taken months for new technology adoption to fail or succeed, results can now be seen in just a few weeks. Hotels are then able to quickly decide whether to fully adopt that new solution or quickly pivot to another technology.
Collectively this will make a significant impact throughout the business, especially in areas like customer service, where technology will be able to make real-time, personalized suggestions for everything from room upgrades to acting like a virtual concierge with dining suggestions.
Artificial intelligence (AI) in general, and generative AI in particular, excel at identifying patterns. However, to achieve this, they require accurate and bias-free data, as well as a well-designed training process. In the context of hotel management, the PMS data model handles critical information about customers, reservations, stays, and billing.
We are currently witnessing a potential decline in the exaggerated enthusiasm for generative AI. Although it is acknowledged that AI will shape the future of society and industries, finding use cases with tangible returns is not as straightforward as expected.
An effective strategy involves identifying use cases that generate clear and quick benefits, thus driving the adoption of AI in our companies. Specific examples could include upselling strategies, using purchase propensity algorithms, and detecting errors in financial audits and billing processes. These use cases would leverage PMS data and could be implemented swiftly and efficiently, promoting business growth and effectiveness.
How will we roll out AI tomorrow morning and this next year? There are two answers here:
1. For training and customer support of complex systems, the operator's guides for each are now able to be fed to any of several existing limited (not large) language models (micro-LLM's) that are restricted to the user property's account. Within minutes or less from creation, any user on the local system is now able to ask natural language questions that might concentrate on a single task or combination of tasks.
2. For complex questions by staff or management, there are now and will be more vendors linking micro-LLM's to accounting systems, reservations and front office databases, CRM, sales department contracts, emails sent and received through the system, messaging from guests and within the property and more. At the speed we are seeing vendors use new tools from major vendors such as Google and Microsoft to create their LLM's, this will mature quickly - perhaps by the time you read this.
Related article by Dave Berkus
Rather than PMS guest profile extraction, I'd focus on your CRM/CDP and social media listening tools and extract guest feedback data from these and use Gen AI tools to create segments around top topics. Your CRM/CDP should be the central place for guest information, not the PMS. Also, download data from third party tools like Tripadvisor and look for patterns, eg feedback on your website, F&B offerings, call centre agents, retail offerings etc. All feedback is useful, and a good use case for Gen AI is to summarise large volumes of data. Share the highlights with staff so they can implement operational improvements.
Behaviors of guests & sorting of all offerings (room, packages, meals, drinks, activities) based on previous behaviors. Much of this if done on a per person can be done using general purpose AI along with a just in time promp injection model. Does not even need machine learning. However, it can use machine learning in aggregate to provide suggestions to new users who have no history with the particular hotelier.
The best data that a PMS stores is its guest history - travel purchase, rooms stayed in, ancillary purchases, number of occupants, special requests and so on. While we can discover some patterns to help grow the business, a machine can sift through this multitude far more efficiently to find patterns and do so in an unbiased manner.
Once you have some pattern recognition in hand, the best use cases are all about personalization:
1). Anticipating special requests: A hallmark of hospitality service, ML can help you to scale instances where incoming guests are identified as wanting particular setups (extra towels, extra pillows, two bottles of a particular mineral water in the room etc.), prompting you to ask them if they indeed would like this to show that you care.
2). Ancillary upselling: This one has been described at length with platforms that are helping augment guests' stays. It works, and it works better when machines can build an initial model off guest history data.
3). Rooms upgrades: Hospitality is also a business of 'surprise and delight', and there's nothing that can make a guest happier than a upgrade, with ML cluing you into which guests deserve or will appreciate this.
PMS data is only one source of first-party data, and it's actually quite limited because it focuses only on past guests. A richer set of data is built when combining PMS with CRM data and direct website behavior data.
Once powered by that dataset, some key practical applications are:
- Benchmarking: create automated competitive sets for each hotel and find opportunities for improvement.
- Predictive Personalization: understand user behavior and respond to it in real time
- Audience creation: find the ideal audience for your hotel, both by segmenting users that come to your website property, and by finding similar, high-value guest.
Over time the value of this type of technical capability wiill increase for the industry.
However, in the immediate state, one of the consistent challenges industry has faced is to better undertand the customer. Initially at a macro level and eventually at a micro level.
Core hospitality systems do track a significant volume of behvioural information, whether that be spend related or preference related. Although generally these system do not provide a depth of analysis. Aggregation exists, but in specific subsets based upon the pre-programmed reports or queries.
This is where opportunity for immediate advantage can be gained withthe broader, more unique or specific review of sets of information. The combination of available data with flexibilty of the type of information output, based upon the ask goes well beyond the standard outputs from operational systems. The power of analysis and prioritisation of information at speed is remarkable.
The back of house presents additional opportunities across maintenance, supply chain and even manpower utilisation.
Used frequently, this type of information can be applied back into the business on a shorter timeframe with targeted results than has been the case using the core metrics, KPI's, processes or tools that industry has traditonally leveraged.
To innovate and boost productivity in 2024, hoteliers should investigate advanced machine learning (ML) applications utilizing data from Property Management Systems (PMS):
1. Predicting Lifetime Value and Segmenting Advanced Visitors:
Application: Create machine learning models to evaluate PMS data with online activity, behavioral analytics, and other data to enable accurate guest segmentation and lifetime value estimations.
Value: Customizing marketing and loyalty plans for valuable customers increases retention through deals on upgrades or special experiences.
2. Optimal Resource Distribution and Flexible Workforce Scheduling:
Application: Use machine learning to optimize staff scheduling and resource allocation by considering expected occupancy and trends in guest behavior.
Value: By modifying workforce levels in real-time to match changing demand, such as expanding concierge services for neighborhood events, it improves operational efficiency and lowers costs.
3. Sentiment analysis for dynamic pricing and personalized marketing:
Application: Use sentiment analysis on visitor reviews to improve marketing and dynamically modify prices.
Value: Enhancing pricing strategies' agility and marketing resonance by reacting in real-time to guest sentiments to modify rates or offer promotions, which increases reservations and satisfaction.
Given the huge amount of data inherent in hotel operations, such as check-in/out dates, guest names, booking sources, pacing, preferences, and market trends, it is imperative to reconsider the strategic use of these resources. Drawing parallels with industry giants like Reddit, Twitter, and Tesla, which have capitalized extensively on utilizing (selling?) their data to train AI models, the hospitality sector should recognize this information as a critical asset—perhaps its most critical. The pivotal question then arises: how can these data be valued and effectively utilized?
The answer involves navigating the complexities of property management systems, which serve as the primary repositories of this data, often constrained by technical or commercial (if not political) limitations. Once these issues are addressed (and we're a looong way), I think the main application of machine learning reveals three promising areas: predictive guest personalization, dynamic pricing strategies, and operational automation.
Fix the data silos problem, and we'll get there.
The future of machine learning (ML) in hospitality applications is set for a transformative shift through readily trained large language models (LLMs) and prompting. This democratisation of technology enables even those with minimal technical skills to enhance hotel services, from personalised guest interactions to efficient back-end operations.
Prompting offers a "no-code" approach for creating AI applications, allowing hoteliers to develop solutions in natural language, bypassing the complexities of traditional programming languages. OpenAI provides capabilities similar to workflow automation tools like Make.com and Zapier, enabling hoteliers to act as developers.
AI needs extensive access to hotel data to make informed decisions and the ability to implement these decisions seamlessly into daily operations. API-first architecture facilitates both effortlessly, driving unprecedented innovation and competitiveness in hospitality.
The most common applications, currently centred around inventory optimisation, revenue management, upselling, guest communication and concierge applications, are set to expand. The simplicity of no-code solutions means that these tools are scalable, adapting to the needs of both small inns and large hotel chains. The future will likely see a spread of "top hospitality prompts" curated by major players in the industry, shared publicly to standardise and enhance AI application across the sector.
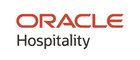
This World Panel Viewpoint is sponsored by Oracle Hospitality
More information